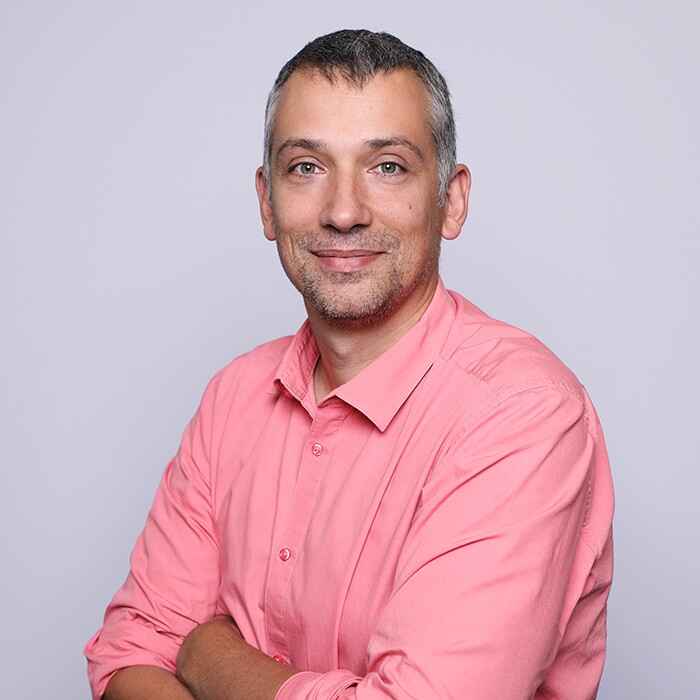
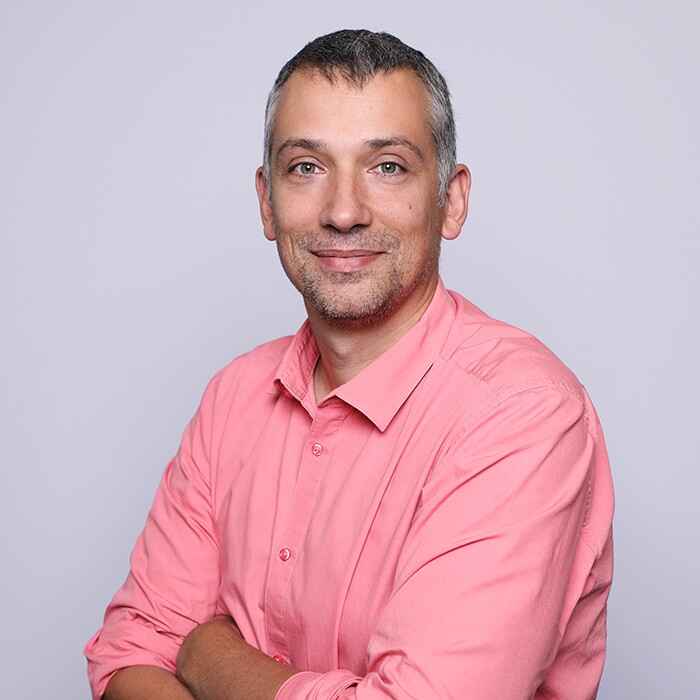
Chatting with the Past
Geschichte ist Gegenwart! Der History & Politics Podcast der Körber-Stiftung
Imagine it is 2023 and you can chat with all the historical figures you have always wanted to talk to. Unfortunately, you can't do this yet, but you can engage with chatbots that claim to be able to imitate historical figures. Artificial intelligence promises to interact with the past in unprecedented interactivity. As AI continuously develops, educationalists warn that pupils and students already use tools such as ChatGPT on a daily basis. In our August episode, we explore with our guest Frédéric Clavert, Assistant Professor in European Contemporary History at the University of Luxembourg, the possibilities and challenges we encounter, when dealing with the past by using 'large language model' tools such as Chat GPT.
Information about our guest
Frédéric Clavert among the guests of ChatGPT, A.I. & History: A Round Table Discussion, organized by The History Communication Institute and the Explorers of the International Federation for Public History, February 2023.
More information on the use of ChatGPT in history education Moira Donovan, How AI is helping historians better understand our past, in: MIT Technology Review, April 11, 2023.
Ludwig Siegele / Oliver Morton, How AI could change computing, culture and the course of history, in: The Economist, April 20, 2023
History Communication Institute, A Statement on Artificial Intelligence, June 2023.
Wulf Kannsteiner, Digital Doping for Historians: Can History, Memory and Historical Theory be Rendered Artificially Intelligent?, in: History and Theory 61 (2023), No. 4, p. 119-133.
Christian Götter, ‘Künstliche Intelligenz’ schreibt künstliche Geschichte, in: Geschichte in Wissenschaft und Unterricht Nr. 5-6 (2023).
Glossary
ChatGPT: ChatGPT, in full Chat Generative Pre-training Transformer, software that allows a user to ask it questions using conversational, or natural, language. (Source: https://www.britannica.com/)
Large language model: A large language model (LLM) is a type of artificial intelligence (AI) algorithm that uses deep learning techniques and massively large data sets to understand, summarize, generate and predict new content. (Source: https://www.techtarget.com/)
Generative artificial intelligence: Generative AI is a type of artificial intelligence technology that can produce various types of content, including text, imagery, audio and synthetic data. (Source: https://www.techtarget.com/)
Artificial intelligence: artificial intelligence (AI), the ability of a digital computer or computer-controlled robot to perform tasks commonly associated with intelligent beings. (Source: https://www.britannica.com/)
Machine learning: machine learning, in artificial intelligence (a subject within computer science), discipline concerned with the implementation of computer software that can learn autonomously. (Source: https://www.britannica.com/)
Cybernetics: cybernetics, control theory as it is applied to complex systems. Cybernetics is associated with models in which a monitor compares what is happening to a system at various sampling times with some standard of what should be happening, and a controller adjusts the system’s behaviour accordingly. (Source: https://www.britannica.com/)
Data mining: data mining, also called knowledge discovery in databases, in computer science, the process of discovering interesting and useful patterns and relationships in large volumes of data. The field combines tools from statistics and artificial intelligence (such as neural networks and machine learning) with database management to analyze large digital collections, known as data sets. (Source: https://www.britannica.com/)
Subscribe to our Podcast!
You find us at Apple Podcast, Spotify, Google Podcasts and in many other Podcatcher Apps.
If you have questions or suggestions with regard to our Podcast, send us an email to: gp@koerber-stiftung.de.
More information about this episode and about the History & Politics Podcast can be found at: Podcast-Website.
Follow us on Twitter. At our KoerberHistory-Twitter-Handle we tweet about the activities of our History & Politics Department.
“I think it’s [ChatGPT] probably a good way to have new pedagogical ways to teach history, that’s for sure. It will probably be something that we must take into consideration, when we address large audiences, but I hope that it will not be the main point of entry to the past for our fellow citizens.”
Frédéric Clavert
Assistant Professor in European Contemporary History at the University of Luxembourg
Weiterführende Informationen
- About Frédéric Clavert
- Frédéric Clavert among the guests of ChatGPT, A.I. & History: A Round Table Discussion, organized by The History Communication Institute and the Explorers of the International Federation for Public History, February 2023.
More information on the use of ChatGPT in history education:
- Moira Donovan, How AI is helping historians better understand our past, in: MIT Technology Review, April 11, 2023.
- Ludwig Siegele / Oliver Morton, How AI could change computing, culture and the course of history, in: The Economist, April 20, 2023
- History Communication Institute, A Statement on Artificial Intelligence, June 2023.
- Wulf Kannsteiner, Digital Doping for Historians: Can History, Memory and Historical Theory be Rendered Artificially Intelligent?, in: History and Theory 61 (2023), No. 4, p. 119-133.
- Christian Götter, ‘Künstliche Intelligenz’ schreibt künstliche Geschichte, in: Geschichte in Wissenschaft und Unterricht Nr. 5-6 (2023).
Just a few months ago, BBC News delivered a powerful headline: “Artificial intelligence could lead to extinction, experts warn”. At the same time, 92 % of people asked in a survey in Germany are already familiar with the term ‘artificial intelligence’. This is no surprise, as this is the topic on newspaper front pages around the world.
However, for most of the interviewed Germans, artificial intelligence carries a very negative connotation, often associated with various fears. Yet, experts tirelessly emphasise that artificial intelligence will change our everyday lives forever. Despite this, we know very little about the effects it has on our historical and political self-image.
For our August episode, we invited Frédéric Clavert to discuss with my colleague Felix Fuhg, programme director of eCommemoration at Körber-Stiftung, the use of artificial intelligence in history education. Frédéric Clavert is Assistant Professor at the Centre for Contemporary and Digital History at the University of Luxembourg. After studying political sciences and contemporary history in Strasbourg and Leeds, and after different academic career steps, he started to become interested in Digital Humanities, when he was working at the former Virtual Centre for the Research on European Integration in Luxembourg. Today, Clavert is one of the leading historians in Europe, passionately exploring the impact of artificial intelligence on our understanding of history. Currently, he is working on an edited volume that delves into the question “Is artificial intelligence the future of collective memory?”.
Today, we particularly look at new opportunities to get engaged with history through the function of a virtual chat. Now, let’s get started with our new podcast episode that will help everyone interested in history education to identify possibilities and limitations using generative artificial intelligence in order to bring people into interaction with the past.
Felix Fuhg: Thank you very much for being our guest today, Frédéric. Let us start with a very personal question at the beginning. Do you remember, what was your first contact with AI technology as a professor for European history in Luxembourg?
Frédéric Clavert: Yes, I do. So, first, thanks for the invitation. It was actually before the University of Luxembourg, it was when I was doing research as a teacher – and now it’s been two years that I’ve been teaching about ‘large language models’. But that’s a hard question to answer in fact, because as historians, we may have all already had contact with AI without knowing it. For instance, if you go to an archive center, you will take pictures of your archive, of your documents, right? And you might take those pictures with your smartphone, and your smartphone has some AI embedded in it. Some sort of AI based software and applications, so we may have all already used AI without knowing it.
As a researcher, what I do is basically using text mining – and it’s been around 10 years now that I have been using text mining, and all text mining relies on AI. I’ll use it for instance, when I use a piece of software called MALLETS, MALLETS is a text mining software that is doing, amongst other things, topic modelling and this topic modelling is based on LTA, which is a sort of topic modelling that is based on machine learning, which is a branch of AI. As a teacher, I teach a Master course called “European Collective Memories in the Digital Era” and there is one session, where we use GPT, GPT is the engine behind ChatGPT, to see how collective memory is embedded in ‘large language models’.
As you already mentioned, Frédéric, AI started to leave a footprint on history in various ways, and it offers new opportunities for researching and reconstructing the past, but also it changes the representation of history in digital times. And when it comes to research, AI tools, for example, make it easier to identify persons in historical photographs. In this episode however, we are particularly looking at ‘large language models’ and their utilisation in uncovering history. So how many people are using ‘large language models’ such as ChatGPT or Bard or Bing, and has its use for your own work with students had any impact on, for example, your marking of papers at university?
How many people are using LLM tools? That I don’t know to be honest, but virtually everybody can use them, because ChatGPT for now surely has a Beta Software, but it can be used by everybody, you create an account and then you can start using it – there are some constraints, but virtually everybody can use such tools. And through Bing, which is based on ChatGPT you can also use these kind of tools. I have not yet been confronted directly with students using ChatGPT for their essays, that is the essays they hand over to me, in my courses. Some other colleagues have been. But I have a Bachelor course next semester that is based on essays written at home, and we are currently thinking, with a colleague, who is doing the same teaching, but in German, how we are going to organise the evaluation of this course.
But, I have an anecdote to tell, still – during this Master course teaching that I was talking about, a few weeks ago, we assessed the quality of some texts produced by ChatGPT about Jean Monnet, he’s a good example, because he’s quite well-known, but not too well-known, I mean, it’s not de Gaulle or Bismarck. So it’s easier to see, where ChatGPT will fail. But, there was this one student and she seemed really unhappy, and I asked her why she seemed so unhappy. And in fact, while working with ChatGPT she realised that ChatGPT has some sort of writing style, something very neutral. And she had a collective essay to write with other students for another course, and was really unhappy with the introduction of this essay, but she could not say why. And in fact, while working with ChatGPT in my course, she realised that this introduction was probably written by ChatGPT, and not by the student, who claimed she wrote it. So she went to this other student and asked her if she had used ChatGPT, and indeed she had used ChatGPT to write the introduction – so she asked her colleague to rewrite the introduction, this time in a more human style.
We’ve now already mentioned tools like ChatGPT, but we also introduced the complicated term ‘large language model’, so could you briefly say what technically speaking a ‘large language model’ is and how it works?
Yes, so what I’m going to do is to explain it the way I’m understanding it, as a non computer scientist, as I am a historian. So ‘large language model’ is basically an AI powered system that is trained, that’s the ‘P’ of ChatGPT, ‘P’ meaning ‘pre-trained’. So that is trained on massive data sets, in the case of ChatGPT this includes for instance the use of ‘common crawl’ a sort of filtered snapshot of the living web. Those ‘large language models’ are mostly based on neural-networks that loosely imitate how our brain functions. It’s a loose metaphor. And neural-networks are able to ‘learn’ from the training, from training data sets. Learning, here, means in fact statistics and probabilities: for each word in the training data set, the AI based system will deduce the probabilities of this word to be associated with other words. The way it is deducing this is not always clear, it’s a bit of a black box.
Today, we speak about ‘transformers’, which is a sort of neural network defined by Google in 2017, and ‘transformers’ are better at taking into consideration the general context of a word within the training dataset. So this is better. The results it produces are better than what neural networks used before 2017. So basically, the texts generated by, for instance, ChatGPT, are texts made of words that are statistically pertinent. Which doesn’t mean that they are pertinent from a human perspective or that there is a notion of truth in them, this is, in the end, complex and hard to understand statistics, but it’s just statistics.
This directly brings me to another question, because artificial intelligence has become a commonly used term, but what are actually the dimensions of the definition, and to what extent is the term and the often used description really linked to a new era of data analytics and data modelling?
Can I start with a bit of history of computing? So, basically there are several families of artificial intelligence, I’m not a historian of computing, but I’ve read about those families. Within those several families of AI, there’s a difference between symbolic and connectionist AI. Symbolic AI imitates the way we reason, we think, insisting on logic – and that was popular in the 1980s, 1990s, but then the results were still quite limited. A connectionist AI imitates, again, loosely, see this as a metaphor, how our brain works. Yann LeCun is one of the leading AI researchers, today working at Facebook, and he says that between neural networks and our brain, there is the same difference as between the wing of a plane and the wing of a bird. So that’s just a really loose metaphor. Today, it’s basically connectionist AI, which imitates how our brain works, that is the most popular, in particular with deep learning through those neural networks. Deep learning is the basis of ‘large language models’ today, but from what I have understood, some ‘large language models’ are based on connectionist AI, but for some elements they are still using symbolic AI, so we are going to have something that is in its majority connectionist AI, but still uses something from symbolic AI. But both families of AI have roots in the 1960’s, if not the 1950’s, and in this sense, it’s nothing new.
In fact, AI is a project that is really part of computing since the beginning of computing sciences. Just think about the famous Turing test that was described in an article in 1950. In this context, some authors, some historians, even evoke the human project of creating automatons that look like us, by artists during Antiquity, at least in the western world.
So you can do a really long-term history of AI in this sense. I think that what’s new is the fact that computers until the 2010’s lacked power and data to make deep learning based applications really efficient. You remember all the hype about big data – and AI, it’s more or less the same thing, or two sides of the same coin. And since the 2010’s, since this hype of big data, basically we’ve got the computing power and huge data sets to make connectionist AI work quite well. In terms of data analytics, data modelling, I’m not sure there is a before and after from the point of view of historians, because as I said, the standard applications that we are using, were more or less here 10 to 15 years ago, MALLET, Latent Dirichlet Allocation for instance, which is the standard topic modelling application today, was defined, if I remember well, in 2008. That’s quite parallel to the rise of digital humanities and digital history, but there’s this thing that is changing with ChatGPT for instance, and there are other LLMs like BLOOM – data analytics gets much easier for different reasons, first, because while the GPT is pre-trained, you can add another training, and you can have a version of GPT that will be a bit more specialised. So if you do a complimentary training with it, you can easily detect persons, dates, etc. So that’s a way to do data mining in the end. There is another thing, which is that, because it is today quite easy to write code, including data analytics code, ChatGPT can really simplify your life. I’m writing code with the help of GPT, because the code is not the core for my research, it just enables it. And it’s pretty efficient, it’s pretty good. And now I’m able to read the code and can be sure that the code really does what I wanted it to do.
I have to say that you’re definitely a proper historian, because historians love to put phenomena and developments into larger context, and that’s what you’ve done now. As you already mentioned, the British Alan Turing, for example, or the cybernetics movement of the mid 20th century, had often been seen as one of the earliest forms of AI development in history, does, in your opinion, historisation help us to understand the current debate regarding the impact of AI on society and the way artificial intelligence is changing the notion of history through its implications?
I think it’s quite important to historicise things. There’s a debate about that, but you could argue that computerisation is just the continuation of the industrial revolution for instance, so in that case you can see the advent of computers in a 300 hundred years context, which helps us understand, particularly when we refer to time, that there is a continuous movement towards automation since say the beginning of the 19th century, but now when we talk about the industrial revolution, we go back to the 17th century basically. So you can see, in this sense the industrial revolution as a movement that was at first quite slow, and that then accelerates – and this helps us to understand, for instance, how we perceive time, there are many, many authors, Reinhart Koselleck for instance, who spoke about time and the fact that we have this sense of acceleration of time – and computerisation in this way is one more step towards this acceleration of time, and that can help us to understand why we see time and history differently today. That is something important, but to be honest, I don’t always like the trend of historians to say ‘that nothing’s new’, in fact historisation also helps us to understand what’s new. And I think that with the networkisation of data, with the emergence of personal computers, of servers, of the web, of networks – I think that’s a sort of rupture, a sort of radical change – and the massive amounts of data that we are producing today, because basically in one year we are probably producing more data than from the beginning of human history to 2010, that’s a big change. That’s really a big change and that enables new things that have some roots in history, but that are still new, and that we need to understand a bit better, including AI and how we delegate, as today we are able to delegate connective tasks to the machine. And connective delegation to the machine is not exactly new, but today it can indeed become massive.
And today, newspapers all over the world are full of articles looking at the transformative path of artificial intelligence and for many experts AI turns the world upside down, especially for those, who traditionally worked analogue, like historians and memory workers, and people working in museums for example – have in your opinion, Frédéric, AI and particularly those tools, which work with so-called ‘large language models’, have those tools the potential to have a not inconsiderable impact on remembrance culture, history, education, and the way we think about history also in the long run?
I think we need to look at several things. First, ‘large language models’ are trained on data sets.
On those famous training data sets, and those data sets are embedding views, visions of the historical past, descriptions of facts – and that we as historians may not agree with. So basically all ‘large language models’ are coming with some embedding notions about the past that can be quite diverse.
And the problem here is twofold, first, commercial ‘large language models’ refuse to give the details of their training data sets, we have a rough idea of those training data sets, ‘common crawl’ that I have already mentioned is basically a sort of snapshot of the web, but it’s not the living web. We know that probably wikipedia has been used, but that’s already in common crawl anyway. And for ChatGPT, there is a supplementary training, if I understood it right, on reddit datasets, reddit is a set of forums on the web, and reddit data sets are good for the conversational aspect. But we don’t know the weights between those different parts of training data sets, so we don’t know any details of the training. And then those data sets are massive, ‘common crawl’ is 80 TB compressed, and it’s mostly text, so the compression is very efficient. Therefore it’s really hard to assess what’s in there and to try to assess what kind of history is in there, inside those training data sets, what kind of visions of the past are embedded in those data sets. That’s the first of two aspects.
The training data sets are an important aspect to consider, and then it’s a question of statistics: the generated text is statistically pertinent. In a way, look at the generated text as an average text. Hence the typical style of ChatGPT that is very balanced and neutral. And last, but not least, this is less documented, in order to avoid answers of ChatGPT that could be inappropriate, that would be racist for instance, there’s a supplementary training through digital labour, so basically humans correct answers of ChatGPT, and ChatGPT will learn from those corrections. There are also biases from those humans correcting the answers from ChatGPT. And even when you’re using ChatGPT yourself, you correct the answers, so that also means interpreting the training data sets of ChatGPT. In that case, for ChatGPT, badly paid Nigerian digital workers were correcting the answers and lots of them refused to work, as it is traumatic work – these are some ethics we should consider.
In the end, the generated texts that you have are average. It can sometimes work, probably better in English, because 80 to 90%, we suppose, of the training datasets is in English. But sometimes this average text is really bad, often in its details – it can look convincing, but most of the details are wrong. So in other words, it produces text that may appear ‘good enough’. But ‘good enough’ is not what historians want to get, right? It’s not what we aim at, when we are doing research, we want to do something more than ‘good enough’, ‘good enough’ is not good enough for historians. Furthermore, in the case of ChatGPT, the data it was trained on dates back to December 2021. It’s not about news, it’s not about advances in historiography for instance. It’s a sort of conservative ‘good enough’, based on data, where the recent past is very much more represented than the past before the web, or even before 2010 – because we are producing more data, it’s a sort of ultimate presentism, based on the present as it was in December 2021. So that’s another problem, there is much more data about someone who lives today than about someone, who lived in the past. So that’s also something important, as that will have an impact on the way we see the past. I hope I have answered your question, because it’s complicated, a complex question.
It is, and as we looked at today’s topic in our first question from a bird’s eye perspective, it seems to me that it makes sense to dig a little deeper into today’s focus topic, namely generative artificial intelligence and Chat GPT and ‘large language models’, something that we call generative artificial intelligence nowadays. And we call it generative artificial intelligence, because it is a form of machine learning that is able to produce texts, video, images and other types of content – for us as users the most well known generative artificial intelligence today is ChatGPT, and to give our listeners a good impression of what ChatGPT is capable of – many of you will probably remember the debate that came up when the Australian historian Christopher Clark published his book “The Sleepwalkers. How Europe went to war in 1914” and the book deals amongst other things with the difficult question, which nations and political powers were involved and responsible for the outbreak of the First World War, and as you will probably know as well, it is still an ongoing debate, if European nations “sleepwalked” into the war. Let us see how Chat GPT answers the difficult question, “who is responsible for the outbreak of the First World War?”
Chat GPT: “The outbreak of the First World War was the result of a complex set of factors and events. While it is not accurate to attribute the entire responsibility to a single party or individual, there were several key factors and countries that played significant roles in the lead-up to the war. Here are some important factors and parties involved.”
And then Chat GPT is mentioning the alliance systems, imperialism and rivalries, nationalism, assassination of Archduke Franz Ferdinand, the failure of diplomacy back in the day and so forth and so forth, Frédéric, what do you think about the given answer of ChatGPT and does the answer match, in your opinion, the complexity of the debate? And what are the challenges in your eyes within the given answer?
The text is not too bad, but it’s a synthesis, so there is no problematisation, for instance, it’s not research of course, it’s not what we expect from our students, maybe at Bachelor, but not at Master level. There is no explicit relationship to current researchers or to current research debate, because it’s a synthesis. So for instance, if I remember well, you mentioned Christopher Clark’s piece, but there are no “Sleepwalkers” in this text, so there is no reference to Christopher Clark, there are no references to those debates. It’s very convincing, the style is very convincing, so it really always looks very current. It’s ‘good enough’ in the end, but the problem for me here is that you have no clue about the sources of this text.
Where did this text come from, you don’t know, it’s a black box, you can ask Chat GPT for references, and it will give you references, but they will be made up. Some of our colleagues on Twitter or Mastodon complain, because they were contacted by journalists, asking them in an interview about their latest article, and the title of the article was consistent with their research, but they never wrote it, and that’s because ChatGPT gave the title of the article, so it was current, but false. I have done something very narcissistic and asked ChatGPT to write my biography or the biography of some of my colleagues and for instance, I asked about a biography of Andreas Fickers, who is the director of Luxembourg Centre for Contemporary and Digital History –
It’s normally totally wrong, isn’t it?
It was current, but for instance, this was, because Andreas is a bit more well-known than me.
It’s always about the question of how popular you are in the end, right? How much information is in the data set about you, right?
And within the references that were at the end to explain what Andreas wrote, there was one of my books, and books from others, so it’s current, because probably one of the C²DH websites is in the training data sets, so there is data about us. It’s just that it’s statistically pertinent to have the title of this book with the name of Andreas, because probably it’s on the page where, with this reference, there was my name and the name of Andreas for another reason.
There is no notion of truth, of false and right, in ChatGPT, because it’s a ‘large language model’, it’s made to produce good language, not to produce something that is false or true or whatever, so there are no references. But this text about the First World War is not bad, because it’s statistically pertinent and because the outbreak of the First World War is quite well documented. But it’s not a text that is satisfying for us and it’s not a text, if it was produced by a student, that would be satisfying when we mark, when we give a grade to students.
In our previous question we used ChatGPT for reconstructing and summarising a so-called research debate, but ChatGPT offers many more opportunities, we can, for example, ask Chat GPT to let Otto von Bismarck comment on the Russian invasion of Ukraine, an event that started in February 2022. Bismarck, as many of you will probably know, died already in 1898, and if Bismarck had to react with a political statement to the invasion, what would he say – this is a snippet from a speech generated and delivered by ChatGPT.
Chat GPT: Ladies and gentlemen, today I stand before you to address the pressing matter of the ongoing war in Ukraine. As an observer of history, it is with deep concern that I witness the conflicts and divisions that plague the Ukrainian land. The struggles faced by the Ukrainian people resonate with the challenges and tribulations my own nation once endured.
Okay, that’s a kind of weird response, isn’t it?
Chat GPT: Thus, it is my duty to offer insights and reflections in the hopes of fostering understanding and, ultimately, a path towards peace.
In preparation for our podcast I’ve stumbled over reports of school teachers, in which they mentioned that they let ChatGPT write such speeches and then analysed and problematised them in their classes. What do you think about this this approach?
For me, the text is just nonsense. The vocabulary doesn’t seem part of the 19th century vocabulary, right? As I said, ChatGPT has been trained on data that’s anterior to the Russian aggression, because the training data sets stop in December 2021, so that’s a subtle time inconsistency, but it’s important. Nevertheless, writing that kind of text and asking students to analyse it, can be interesting, pedagogically speaking, to show them, how bad that kind of text can be, even when it looks consistent and current, and from there you can, even if you always need to be present, ask them to criticise, to make a critique of the text, and then you can have them start thinking about how we write history, that’s basically what I do. It’s good training for historical criticism. As a teacher you will have to find subjects, where the answers will be a bit better, and subjects, where the answers will be bad, as that’s also a way to think about biases that are embedded in those systems, but that also reflect the way we see collectively, not as a historian looking at history and the past, and how we will write history. And it’s also a way to think about data inequalities in the world, because some regions of the world lack data about their history, I mean online, digitised data, including large parts of Africa. So I think it’s a nice way to to think about all those matters, so yes, it can be used in teaching.
Another AI tool that is using LLM technology is an app called ‘Hello History’ and the creators advertise the app with the words “bringing history back to life”. The app uses AI technology to create an interactive chat experience that allows people to engage and learn from historical figures, it uses virtual coins, with which users can unlock historical figures such as Gandhi, Marilyn Monroe, Frida Kahlo and many more in order to chat about historical events and developments, but also about recent political and social challenges – and in the last question we looked at the problem already of bringing historical figures back to life to comment on current and ongoing happenings, which are taking place a long time after their death.
One of the key problems in my opinion here is of course that the data mining and the system are based to a high degree on text written by and about historical actors, but what happens in between their death and contemporary events and from which decade of a person’s lifetime does a representation of an opinion gets extracted in the end, since as we know from ourselves, we can easily change of course also our opinions.
On Twitter for example, there was a big debate about these kinds of responses that were coming out of the chatbots, because people posted many examples of how ‘Hello History’ chatbots faked history in the end. One user for example asked Nicholas the second of Russia “Why did you promote ‘The Protocols of the Elders of Zion?’” and the chatbots response was: “I did not promote ‘The Protocols of the Elders of Zion’. In fact, I publicly denounced them and declared they were an antisemitic work that had nothing to do with Russia’s Jewish population.”
Could you please comment on the answer given by the chatbot and explain to us what the problem is, probably within the system that the chatbot is giving us such a wrong response in the end?
So the answer is historically false, it’s as simple as that, there’s not a single word that is true, it’s not even plausible fiction. It’s not even storytelling, it’s just, no – no way this answer would reflect history.
Basically, it‘s based on GPT, so the same engine behind ChatGPT. They do not say if they have done a complementary training of GPT, if they have optimised it for history, and if they did, with which data. It’s a black box. But basically, it’s in GPT, and there might be some explanation why there is this response in this case, because, you don’t want antisemitic answers, when you develop a ‘large language model’. So that may be an explanation of the answer.
If I can explain a bit more, in all honesty, I was quite puzzled when I looked at this website, this application: they mix fictional and real characters, they provide images of those historical persons that are probably generated by image generation systems, and if you look at the details of an image, it’s so inconsistent – Gilgamesh’s or de Gaulle’s portraits are quite interesting, because the uniform of de Gaulle has never existed, is not plausible and does not even look French, I’m not a military historian, so maybe I’m wrong, but it does not even look French! And Gilgamesh is portrayed as a body building world champion, so that’s not consistent with anything. It’s really weird, so it would be even more interesting to see why they selected those real or fictional characters, how did they choose those images – that could be a nice Master’s thesis for a student to be honest!
Nevertheless, asking students to work on ‘Hello History’ can be a good pedagogical exercise: why those characters and not others? How did they choose them? Why this focus on ‘great’ people of the past? Whether they are fictional or not. Assessing the quality of the answers, trying to get information about the system, how it works, etc. all that can be done in teaching. As I said, it could even be a quite interesting Master’s thesis in public history. Furthermore, it could be interesting to test a version of a ‘large language model’ – I would prefer BLOOM, because it’s public, open source – or of image generation systems (stable diffusion, because it’s open source) that would be optimised for history, and see how people use it, and from the prompts they write for instance, we could also see how people are interacting with history, that would be a way to get primary sources, about how our fellow citizens’ past, that could be very interesting. So, there are things to do. But to be honest, not the way ‘Hello History’ is doing it.
It seems to me that generally speaking, AI technology contributes to a trend we already observe for many years now, something we normally linked over the last couple of years to the practice of fake news – boundaries, that’s also something that you’ve mentioned in your last answer, boundaries between fact and fiction are becoming more permeable. So in public, scholars, experts and tech entrepreneurs themselves call for regulation, probably also because of this, and the History Communication Institute, a leading institute focussing on the influence of technology on our understanding of the past, raises in their thought-provoking statement on Artificial Intelligence the important point that discussions about AI should encompass commitments to its integration in education, rather than solely call for more regulation. Do you agree with this? And how would you recommend using tools such as ChatGPT in teaching history at schools and universities?
I agree with the HCI statement, for several reasons, including the fact that lots of calls for regulation are hypocritical, not really honest. When there is a group of people, asking for 6 months of pause in the research in AI, and when Elon Musk is signing it, that’s hypocritical, what Elon Musk wants is to gain time for commercial purposes.
You know I’m a French citizen, so we sign petitions and when we sign up, the people you are signing with, it’s almost more important than the text you are writing – in this case, when you look at people signing, signing these kinds of calls for regulation, it’s really people I don’t want to sign with to be honest. So that’s the first reason. I think we should focus on critical thinking, because it’s one of the cores of our work, in the end, we’re quite good at that. As historians, we really should stimulate critical thinking amongst students, which is something we do every day. That would be critical thinking in regard to what AI is, and critical thinking about what’s a document today, what’s a text today, the complex relation between text, image, veracity and truth. That’s the things we can do, and we already do in fact, but maybe not with LLMs, but LLMs can be used for that too.
And the policy statement we looked at also asks for strong cooperation between historians and tech companies developing AI tools, often used by the public and by students, but also by everyone with a genuine interest in getting information on historical events and figures, as well as an explanation of historical developments. Why is this necessary? And what issues of current AI tools such as ChatGPT can this cooperation counterbalance?
I think this cooperation is necessary – while I do not fully agree, the HCI statement, it’s not only cooperations with commercial firms, it’s also about cooperating with researchers, with public researchers in computing sciences, I’ve evoked already BLOOM, a ‘large language model’, which is a cooperation between computer scientists and ‘Hugging Face’, which is a sort of French, but US-based, start-up that is quite important in AI today – that’s the way we should do it, we should work with our colleagues in computer sciences a bit more, that’s for sure. This is where we can add something important, the tech companies and sometimes our colleagues in computing sciences, they don’t have a clue about what’s a primary source, and basically training data sets are primary sources. They’re just trying their systems on data sets that just look for massive data sets and that’s it, their point of view is to have lots of content, I’m saying that and it’s a generalisation and some critics are far better regarding this point, but usually they have no clue about the content of the training data sets, and in the end, the big tech company, they don’t care about the content of the training data sets. Some of them, once their system is trained, then they start thinking about biases, and they should think about biases, when training the data sets, so that’s where we could help. We could help those companies, in the private and also in the public sector, to understand how to assess training data sets, how to balance them, how to perform a critique of their data sets. And that’s a second small disagreement with the HCI statement, we should not be too self-centered – anthropologists, literature researchers, sociologists, political scientists, lawyers have a lot to say, too. We should really see this as an interdisciplinary perspective, all humanities and social sciences should be involved in the design of artificial intelligence systems. In some domains of the tech industry, that’s already the case: Ubisoft, in their Montréal branch, has worked with historians for ‘Assassin’s Creed’. What’s possible for the video game industry, should be possible for the AI industry.
Unfortunately Frédéric, when I have a look at the time, we have to come to an end, and we have talked about Chat GTP, and its spin-off product historical figures, and it seems to me that it’s just fair, to give the system also the chance to express its opinion on our History and Politics podcast, and I’ve chosen, of course, the great Charlie Chaplin to say what he thinks about the podcast we produce, so Charlie, “what do you think about the History and Politics podcast of Körber-Stiftung?”
Chat GPT: “Well, as someone who has lived through some of the most tumultuous political times in history, I think it is important to stay informed and educated on politics and world events, podcasts like Körber-Stiftung’s History and Politics can be a great resource for learning about different perspectives and historical contexts that shape our current world. It’s always fascinating to learn about how the past events can continue to impact our present-day political climate.”
So ‘Hello History’ is not able yet to imitate the voices of its historical figures. Although other projects such as the AI driven digital Albert Einstein clone, produced by algorithms, show that technically the imitation of voices of historical figures is already possible. So Charlie’s voice was, as the other given answers in this podcast, produced with AI voice over technology.
We have touched on various challenges of teaching history with AI and also looked at possibilities and certain necessities for giving historians the opportunities to play an active role in the development of AI tools and AI systems – we hope that we could deliver food for thought for all of you, thanks Frédéric, for sharing your expertise and of course thanks for listening out there, we hope to see you again for the next episode of our History and Politics podcast. The last words however are yours Frédéric, our podcast normally ends with a short wrap up, all things considered, is ChatGPT paving the way for new forms of getting into interaction with the past?
I think it’s probably a good way to have new pedagogical ways to teach history, that’s for sure. It will probably be something that we must take into consideration, when we address large audiences, but I hope that it will not be the main point of entry to the past for our fellow citizens.
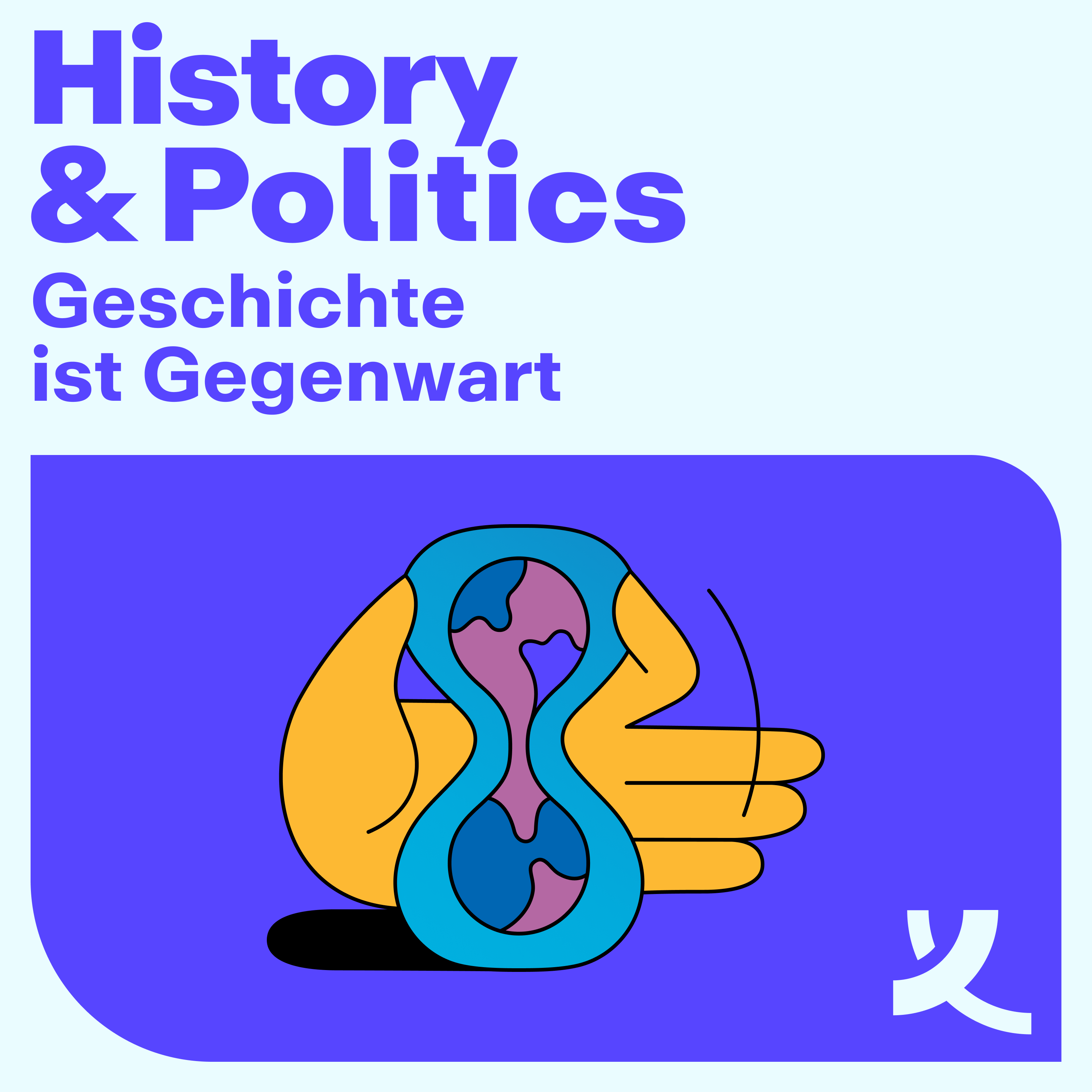
Geschichte ist Gegenwart! Der History & Politics Podcast der Körber-Stiftung
Warum Geschichte immer Gegenwart ist, besprechen wir mit unseren Gästen im History & Politics Podcast. Wir zeigen, wie uns die Geschichte hilft, die Gegenwart besser zu verstehen.
-
The New Germany S03E06: Extremism: Defending Democracy in Past and Present#72 50 Min. 9. Apr 2024
-
Über die Geschichte einer gewaltfreien Gesellschaft#71 45 Min. 2. Apr 2024
-
The New Germany S03E05: Social Transformation: What is German?#70 53 Min. 26. Mrz 2024
-
The New Germany, S03E04: Made in Germany: Industry and Deindustrialisation#69 49 Min. 12. Mrz 2024
-
History in the Age of Disinformation#68 51 Min. 5. Mrz 2024
-
The New Germany, S03E03: Germany and the US: Cowboys and Keynesians#67 51 Min. 27. Feb 2024
-
Distance and emotion: Ukrainian historians in war times#65 53 Min. 20. Feb 2024
-
The New Germany, Season 3 - Episode 2: Germany and France: A Marriage on the Rocks#64 56 Min. 13. Feb 2024
-
The New Germany, Season 3 - Episode 1: Crisis Chancellors#63 55 Min. 30. Jan 2024
-
Technologien gegen das Vergessen#62 36 Min. 27. Jan 2024
-
Amerika – das Ende eines Traums?#61 64 Min. 12. Dez 2023
-
The New Germany, S02E07: Grenzen überwunden? Das Verhältnis zwischen Ost und West#60 84 Min. 14. Nov 2023
-
Zwischen Moral und Realpolitik: Das deutsch-israelische Verhältnis#59 46 Min. 12. Sep 2023
-
Chatting with the Past#58 47 Min. 8. Aug 2023
-
Die Arktis – Von der Friedenszone zur internationalen Konfliktzone#57 37 Min. 4. Jul 2023
-
Zeitenwende and the Return of History#56 44 Min. 8. Jun 2023
-
Polen und Europa seit 1989#55 33 Min. 30. Mai 2023
-
The New Germany, Season 2 - Episode 6: German Politics in Flux#54 43 Min. 9. Mai 2023
-
The New Germany, Season 2 - Episode 5: German Schuldenangst#53 46 Min. 25. Apr 2023
-
The New Germany, Season 2 - Episode 4: Germany’s Grand Strategy#51 48 Min. 11. Apr 2023
-
Politik unter Einsatz des Körpers#52 42 Min. 6. Apr 2023
-
The New Germany, Season 2 - Episode 3: Germany and Poland#49 47 Min. 28. Mrz 2023
-
The New Germany, Season 2 - Episode 2: Germany and China#48 51 Min. 14. Mrz 2023
-
Das Jahr 1923 und German Schuldenangst#47 40 Min. 7. Mrz 2023
-
The New Germany, Season 2 - Episode 1: Germany’s Russians#46 54 Min. 28. Feb 2023
-
Rechte Geschichtsbilder im Video-Gaming#45 47 Min. 15. Feb 2023
-
Asylpolitik: Eine Konfliktgeschichte#44 32 Min. 12. Jan 2023
-
Aktivist:innen in Russland: Zwischen Untergrund und Friedensnobelpreis#43 35 Min. 6. Dez 2022
-
Das Ringen um die neue Weltordnung#42 43 Min. 21. Nov 2022
-
Ukraine: Eine Nation unter Beschuss#41 45 Min. 21. Okt 2022
-
Wohnen – wo Privates politisch ist#40 32 Min. 6. Sep 2022
-
The New Germany, Part 4: The Lid of History#39 52 Min. 22. Aug 2022
-
The New Germany, Part 3: German Energy Policy and Russian Gas#38 43 Min. 8. Aug 2022
-
The New Germany, Part 2: A Love-Hate Relationship#36 57 Min. 25. Jul 2022
-
Russia: When History Becomes a Weapon (Englische Folge)#34 40 Min. 19. Jul 2022
-
The New Germany, Part 1: The Bundeswehr and Germany's Mindset#35 42 Min. 12. Jul 2022
-
Erinnerung hat Konfliktpotenzial – Wie sich unser Blick auf Geschichte ändert#33 48 Min. 7. Jun 2022
-
Krieg in der Ukraine: ein historischer Wendepunkt?#32 35 Min. 31. Mai 2022
-
Geschichte und Erinnerung in Games#31 28 Min. 3. Mai 2022
-
Belarus: Was bleibt von den Protesten 2020?#30 30 Min. 8. Mrz 2022
-
Sind Russland und China heute Imperien?#29 39 Min. 8. Feb 2022
-
#30PostSovietYears: Kann Kunst versöhnen?#28 39 Min. 30. Nov 2021
-
China – Modernisierung zwischen Isolation und Öffnung#27 39 Min. 28. Okt 2021
-
Deutschland und Polen - Geschichte und Zukunft einer guten Nachbarschaft#26 42 Min. 23. Sep 2021
-
Russland - Großmacht mit historisch gewachsenem Sonderstatus?#25 33 Min. 24. Jun 2021
-
Belarus – Über Proteste, Demokratie und Diaspora#24 39 Min. 29. Apr 2021
-
Die Vorgeschichte der Hohenzollern-Debatte. Adel, Nationalsozialismus und Mythenbildung nach 1945.#23 54 Min. 25. Mrz 2021
-
Jüdisches Leben in Deutschland seit 1945#20 50 Min. 25. Feb 2021
-
Deutschland und Frankreich – Fremde Freunde?#22 40 Min. 27. Jan 2021
-
Geschichtsvermittlung ist eine Zukunftsbranche#21 27 Min. 17. Dez 2020
-
Tatjana Tönsmeyer: Das europäische Erbe der NS-Besatzungsherrschaft#19 34 Min. 26. Nov 2020
-
Natasha A. Kelly: Rassismus und die Geschichte Schwarzer Menschen in Deutschland#18 40 Min. 19. Okt 2020
-
Sandra Günter: Sport macht Gesellschaft?#17 37 Min. 24. Sep 2020
-
Stefanie Middendorf: Geschichte der Staatsverschuldung: Kredite für die öffentliche Hand#16 40 Min. 27. Aug 2020
-
Helene von Bismarck: Großbritannien und die EU – ein historisches Missverständnis?#15 34 Min. 27. Jul 2020
-
Aleida Assmann: Was hält Europas Sterne zusammen?#14 24 Min. 25. Jun 2020
-
Birte Förster: Rolle rückwärts bei der Gleichberechtigung?#13 29 Min. 8. Jun 2020
-
Till van Rahden: Demokratie in Krisenzeiten#12 30 Min. 6. Mai 2020
-
Elke Gryglewski: Alles gesagt, alles gezeigt?#11 23 Min. 17. Apr 2020
-
Frank Uekoetter: Pandemien und Politik#10 25 Min. 27. Mrz 2020
-
Mary Elise Sarotte: Die NATO-Osterweiterung.#9 29 Min. 20. Nov 2019
-
Werner Plumpe: Die Kraft des Kapitalismus.#8 21 Min. 23. Okt 2019
-
Ilko-Sascha Kowalczuk: 30 Jahre Mauerfall – und nun?#7 23 Min. 1. Okt 2019
-
Claudia Weber: Kriegsausbruch 1939#6 24 Min. 11. Jul 2019
-
Jason Stanley: Faschismus damals und heute#5 18 Min. 4. Jul 2019
-
Philip Murphy: Rule Britannia?#4 24 Min. 27. Jun 2019
-
Hedwig Richter: Volksbegehren oder Staatsgewalt?#3 22 Min. 20. Jun 2019
-
David Ranan: Israelkritik und Antisemitismus#2 22 Min. 13. Jun 2019
-
Eckart Conze: Von Versailles 1919 zu den „Neuen Kriegen“#1 28 Min. 28. Mai 2019